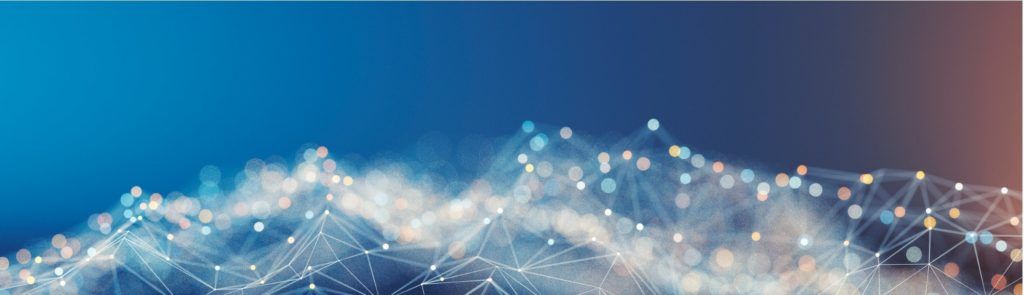
We started Feature Labs with a straightforward goal: build products that enable any organization to create and deploy machine learning solutions.
In 2015 while working in the MIT Computer Science and Artificial Intelligence Lab, my co-founders and I developed an automated data science system that outperformed 615 of 906 top human teams in three worldwide data science competitions.
The results were so compelling that we decided to endeavor to make the same powerful machine learning solutions available to any organization. Since then, we’ve worked tirelessly with an initial group of commercial customers to achieve this goal. Today, we’re excited to announce the public launch of a set of tools and APIs for automated feature engineering alongside $1.5M in seed funding from Flybridge Capital Partners, First Star Ventures, and 122 West Ventures.
Automated Feature Engineering
Feature Labs was founded to commercialize Deep Feature Synthesis (DFS), an algorithm for automated feature engineering. As an MIT graduate student, I developed DFS to accelerate and optimize the manual, time-consuming, and error-prone process required to build ML models.
Soon enough, the numbers started to support that objective: we published an academic paper detailing our success in competition against nearly 1000 human data scientists. MIT News covered our work, which led to over 200 media outlets around the world sharing our creation.
Validating the Hype
This gave us the visibility we needed to engage with nearly one hundred companies and hear about their struggles to successfully deploy machine learning products. It also gave us unique insight into the critical problem most companies encountered while building ML-based solutions: they treated the process like a research project. Consequently, projects frequently led to proof of concepts or feasibility reports rather than deployed models that actually impacted their business.
The dialogue between Feature Labs and these companies led to many of our first enterprise customers, confirming our beliefs about the market potential. Given the hype surrounding ML and AI in the market, we had many options to consider. Instead of embracing the media and investor frenzy towards AI, we decided to focus on creating a product that could truly deliver high-performance ML models.
After over a year of bootstrapping, growing the team, and validating our technology, we were ready to attract outside investors. After meeting Chip at Flybridge, we knew we had found the right partner to help us expand. So of course, we were excited by his offer to lead our round and join our board of directors. However, after we closed that funding round, we realized that Feature Labs wasn’t ready to publicly launch just yet. Because we had set our sights on a higher bar.
Setting a Higher Standard
From the beginning, we could see the problem in the market wasn’t that ML didn’t work, but that companies didn’t know how to leverage it.
As a result, we had high standards for our product validation. We wanted an enterprise to use it on (1) a business problem never solved before, (2) with a dataset never utilized for ML, and most importantly, (3) by a team that was new to developing and deploying ML-based products. To test practicality and viability, we wanted our solution to be deployed into production and drive business results within 8 weeks of starting the project.
A Milestone for Machine Learning
Across the board, our product continues to demonstrate its immense potential and is already producing a substantial impact across industries. We recently shared how Spanish bank BBVA is using our product to improve the accuracy of their credit card fraud predictions, resulting in millions of dollars of savings.
This past summer, we launched an open-source project called Featuretools with funding from DARPA. The goal of this initiative is to share our vision by giving developers the chance to experiment with automated feature engineering on new machine learning problems. We’re seeing great traction as developers contribute and enjoy a glimpse into what we work on everyday in our labs. MIT is also utilizing the same library to teach 1000 professional learners every quarter through their Office of Digital Learning.
Looking ahead
We’re extremely grateful for the support of our new investors and DARPA as we continue to expand and build out our go-to market operations team with proven commercial expertise. I am also very pleased to welcome John to our team as our COO. John’s expertise is just what we needed as we look to grow and scale the organization with global enterprise experience and proven operational leadership. Feature Labs will keep striving to meet market demand and scale in key areas like engineering, customer support, marketing, and sales.
Thanks for joining us on the journey so far. We appreciate all of the interest in Feature Labs. This is just the beginning, and we can’t wait to show you what we’re working on next!