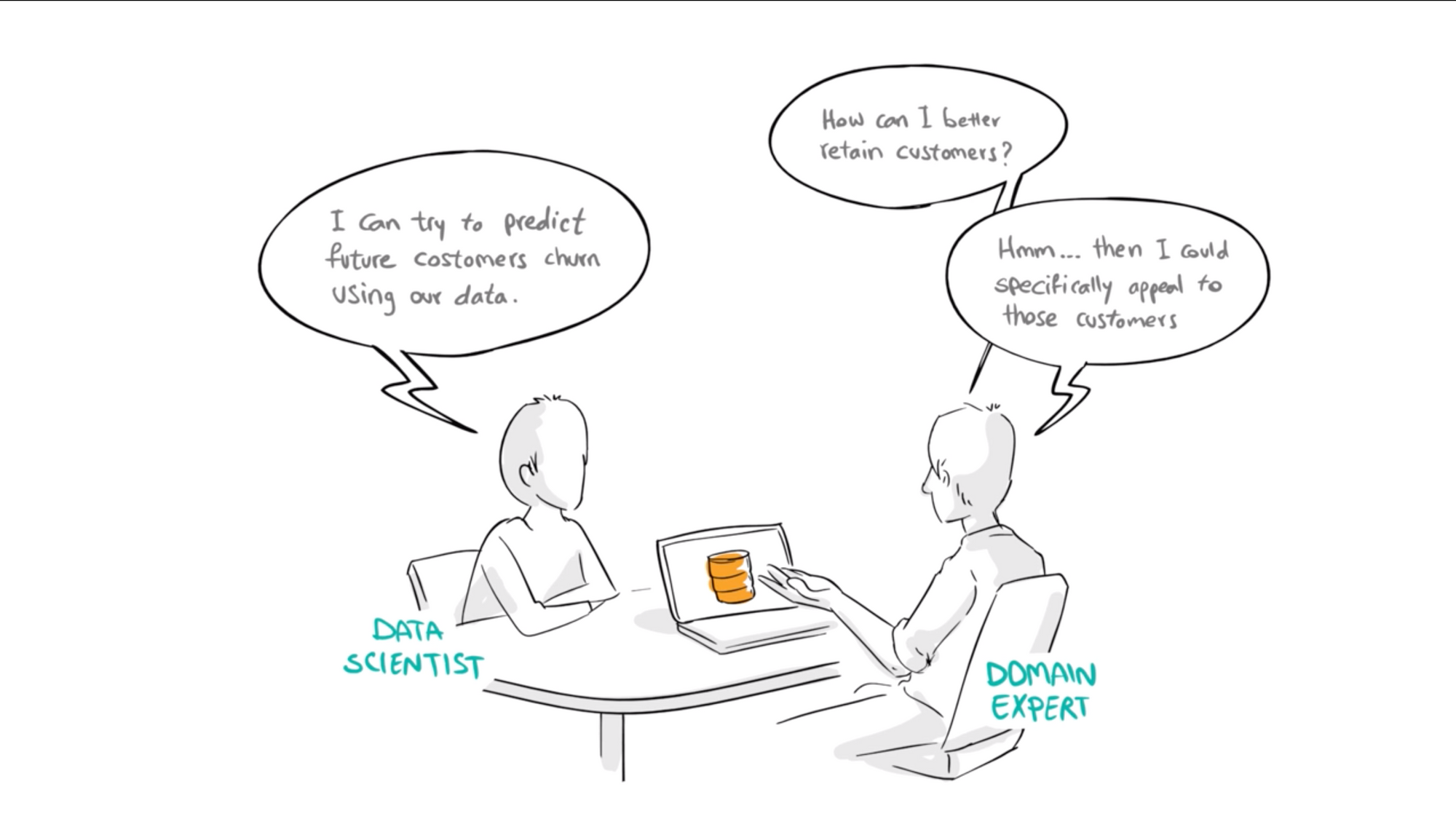
In this paper, we designed a formal language, called Trane, for describing prediction problems over relational datasets, implemented a system that allows data scientists to specify problems in that language. We show that this language is able to describe several prediction problems and even the ones on Kaggle- a data science competition website. We express 29 different Kaggle problems in this language. We designed an interpreter, which translates input from the user, specified in this language, into a series of transformation and aggregation operations…
Published in: IEEE International Conference on Data Science and Advanced Analytics 2016