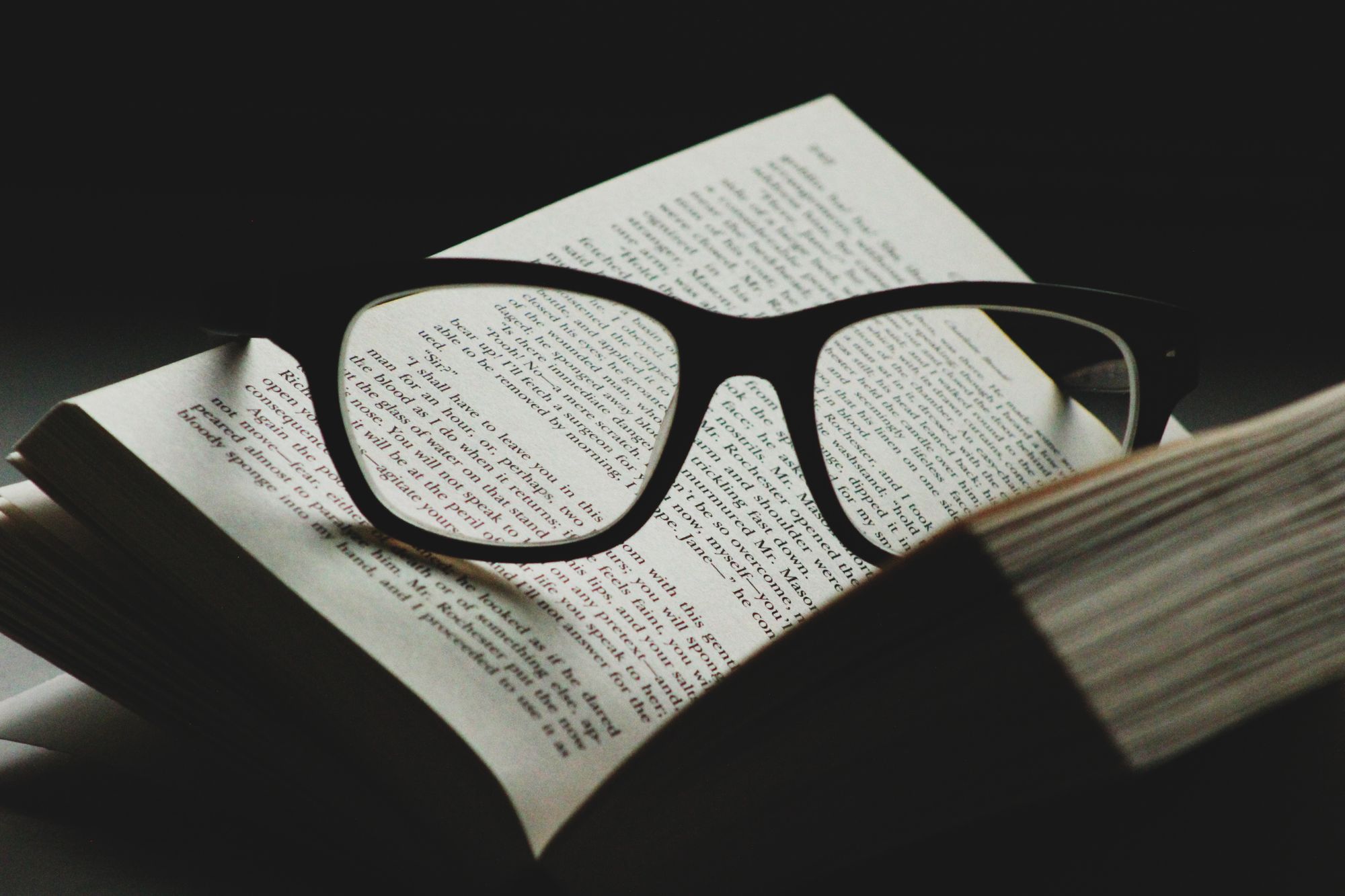
Feature Labs visited Carnegie Mellon University this past July to participate in the 17th annual Simon Initiative’s LearnLab Summer School on Educational Data Mining. During the program we introduced teams to Featuretools, our open source feature engineering library. You can find the complete details in the Featuretools blog post, but here the highlights:
- Teams of subject matter experts in education, who were not necessarily python programmers, were able to prepare their own data for machine learning in less than a week through the Featuretools framework.
- Rather than spending time brainstorming features, scientists focused their time on picking the right problem to solve. This inverts the classical workflow and is important, because the people working on the problem are better suited to thinking critically about what they want to solve than programming a baseline solution.
- Participants were more willing to trust the results than results coming from neural nets. Part of this is because features generated are interpretable, but a larger part is that experts felt they had control over the refinement and interpretation process.
We had a great time and are excited to use some of the things that we learned in similar events. If you want to host a data science workshop of your own, or have data you want to apply machine learning to, please reach out to us at our contact page.